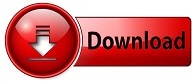
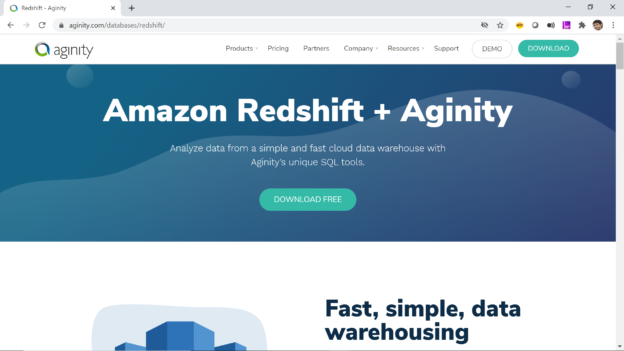
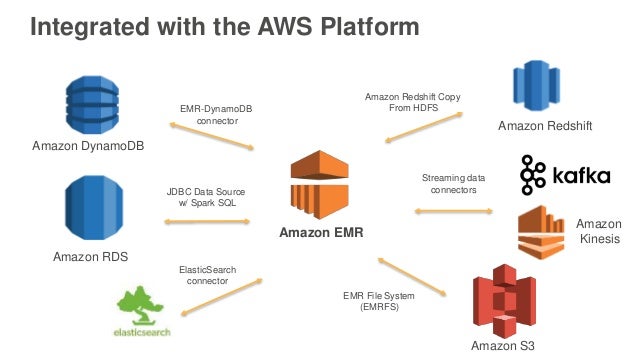
All of these methods are complicated by noise in the data and other data quality issues.

They use statistical approaches, visualizations, and other methodologies to validate claims and identify trends. When claims arrive at the automaker and/or supplier, a team of warranty analysts manually review them. Today, automakers have amassed millions of historical warranty claims dating back to the early 2000s. In many cases, agreements made between automakers and suppliers mean that the cost of warranty repairs on defective parts is shared between both parties. It also includes a text description of the problem.Īfter the repair is complete, the dealership submits the claim to the automaker for repayment where it is reviewed, adjudicated, and stored in a database for historical purposes. In addition to a basic root cause analysis, this document includes relevant details about the vehicle, such as its make, model year, manufacturing plant, cost of repair, and labor required. At the dealership, a technician reviews the problem, diagnoses the root cause, fixes the issue, and then records the findings in a warranty claim document. If one of these 30,000 components breaks during the warranty period, the owner brings the vehicle into a dealership for repair at no cost to the owner. Typically, when a consumer buys a car, it comes with a warranty for defect repairs for a set period of time, usually within 3 years or 36,000 miles from the date of purchase. Most modern vehicles have over 30,000 individual components that are produced by suppliers across the globe. airbags, seatbelts, tires, transmissions) are produced by one or more suppliers, they are assembled into systems, integrated into a complete vehicle, and then delivered to the consumer. Every automaker has its own version of this process, but an example of the general flow is shown in Figure 1. This is because automakers and suppliers must produce parts at very high volume in order to meet worldwide demand.īefore we discuss the specific machine learning model, let’s take a look at how parts are made. Although the advanced risk warning can range from days to months, it’s worth noting that even one week of additional advanced notice can potentially help our customer make significant savings in terms of reduction in faulty inventory and repair costs. With machine learning, a customer can learn from historical patterns leading up to a major product defect or recall, and use a model to predict parts that are more likely to become major product defects or recalls before they happen.
#REDSHIFT AWS PRICING MANUAL#
These methods are largely manual and time consuming. They use heuristics and statistics to analyze patterns, determine the core issue, and then implement the fix at the manufacturing, engineering, or design level. When a recall is issued or a major defect emerges, automakers and suppliers’ work quickly to identify the root cause.
#REDSHIFT AWS PRICING HOW TO#
We explain how to back test the machine learning model to demonstrate how the timing of predictions from the machine learning model compares to the timing of existing quality control processes to quantify how much business value can be realized by using outputs from the machine learning model instead of existing processes. The goal of this post is to explain how automakers and suppliers can potentially use machine learning to help them predict recalls (and major defects) and implement mitigations faster with the aim of reducing the financial impact of these recalls or defects. A recall is the most severe type of warranty claim that is issued when a manufacturer or the National Highway Traffic Safety Administration (NHTSA) determines that “a vehicle defect creates an unreasonable safety risk or fails to meet minimum safety standards.” In the case of a recall, an automaker must replace the defect at no cost to the consumer, and for this reason they set aside large sums of money to cover these costs. In 2021, automakers spent $45.9 billion on warranty claims. Although we focus on automotive, this method could also be applicable to other industries with similar recall use cases. Specifically, we show how predictions generated from an LSTM may provide customers with early indicators that generally out-perform existing manual processes and can help assist our customer’s analysts and engineers to implement mitigations faster with the aim of reducing defective inventory, product repair, and other associated costs. This blog focuses on a potential method to use Long Short Term Memory (LSTM) machine learning models to help customers predict parts that are likely to become defects or recalls.
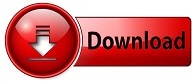